Bias and Error
When AI is biased
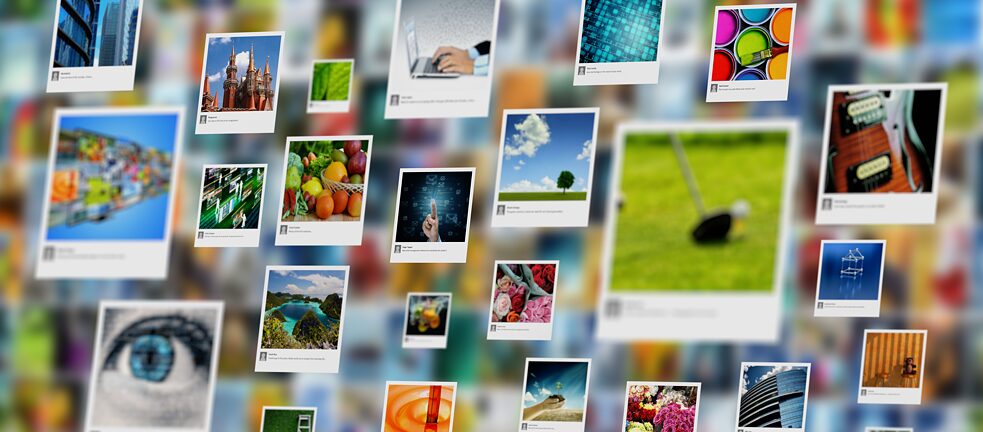
Over the past decade, we have come to spend much of our lives in the digital sphere – a sphere that is increasingly controlled by just a handful of corporations. These companies, unavoidable for most, exert a considerable amount of control over what we can see and say, as well as the types of tools available to us.
Von Jillian C. York
When it comes to online imagery, this control is exercised in a few key ways: First, in terms of what we can see. Companies – and governments – restrict various types of content, from the nude human body to images or videos that contain private information. Take, for instance, Instagram’s prohibition on sexually explicit content or Twitter’s recent rule that a private video cannot be shared. These restrictions, while justifiable, can have a negative impact on the people who use such platforms and may have a legitimate reason for what they are sharing.
Second, popular platforms like Snapchat, Instagram, and TikTok offer filters that distort our images – and often our self-image. These filters, which have been heavily criticized by lawmakers, psychologists, and others for their effect on body image, present us with an often uniform perception of what we should look like. As this perception becomes widespread, it can allow a certain expectation of what we should look like to take hold, potentially enabling discrimination or bias against those who choose to opt out.
Third – and perhaps most troublingly – is the way in which companies use algorithms to serve up content in searches or in our feeds. The effect that this has on the classification and presentation of images in particular is insidious: Algorithms routinely classify images in ways that are discriminatory, biased, or just plain wrong and can have far-reaching consequences for the people who use the platforms that employ them.
Algorithms routinely classify images in ways that are discriminatory, biased, or just plain wrong.
Take, for example, a 2015 incident in which Google’s image recognition technology erroneously classified Black people as gorillas. While the incident was ostensibly unintentional, it is exemplary of how algorithms can be fed training data that serves up problematic results. Algorithms may be purely mathematical, but the data that is fed to them is created by humans, who bring their own biases or ignorance to the table. Furthermore, machine-learning algorithms usually operate as black boxes and do not explain how they arrived at a particular decision – leaving users unable to understand whether such an error was the result of deliberate racism being programmed/built into the code or simply a poorly crafted dataset. And since companies do not generally share the basic assumptions that underpin their technology and datasets, third party actors are unable to prevent such mistakes from occurring.
While stories like this can be easily exposed, the effects of the expansive use of artificial intelligence tools for moderating user-generated content is more difficult to uncover, for we cannot see the vast majority of errors such technologies make, let alone the inputs that cause them.
As former content moderator Andrew Strait wrote in the recently released volume Fake AI, “Notoriously bad at identifying the nuance and context of online speech, these systems routinely fail to identify whether a video constitutes illegal copyright infringement or lawful parody, or whether a post with a racial slur is written by a victim of a hate crime or their assailant.”
The Blind Eye of AI
One pertinent example that has been well-documented, however, is the harm generated by the use of artificial intelligence to classify and remove extremist and terrorist content – and imagery in particular. Over the past few years, in an effort backed by governments around the world to eradicate extremist and terrorist content, platforms have increasingly come to rely upon machine-learning algorithms to detect and remove content that fits that description. But the classifiers utilized are often binary in nature and therefore leave little room for context: If an image contains symbols related to a known terrorist group, it will be classified as terrorist content – even if the reason for the symbol’s presence is artistic or in protest of the group, for instance. Similarly, content that is documented for historic, archival, or human rights purposes will still be classified and likely removed. Relying on technology for such a nuanced task ensures that results will be blunt, leaving little space for essential expression.
Whether it is being used for searches or automated content moderation, artificial intelligence is only as useful – as intelligent, one might say – as its underlying datasets. Datasets that are prone to human error and bias. Therefore, in order to counter discrimination stemming from data, we must be able to peer behind the curtain so that we can understand – and counter – the underlying assumptions and biases of the humans creating the datasets that increasingly dictate what we see and how we see it.
But while transparency allows us to better understand the problem and counter specific errors, we as a society must start asking bigger questions about the role we want these technologies to play in guiding our view of the world. In order to do so, we must stop seeing AI as neutral and begin to understand the inherently political nature of its use.
Whether it is being used for searches or automated content moderation, artificial intelligence is only as useful – as intelligent, one might say – as its underlying datasets. Datasets that are prone to human error and bias.
Using AI to counter extremism serves as a salient example of this. The policies that underlie the use of AI in this context are unmistakably political – they are, to put it bluntly, divisive, separating acceptable (state) violence from that of (certain) non-state actors. While there is just cause for removing violent content from view, the underlying policies are not just concerned with violent imagery, but also with anything connected to a group designated by a company or government as extremist. The end result is therefore not merely the mitigation of harm, but total erasure.
AI is never neutral and its use is inherently political: Why is the content of terrorist groups removed from view instead of violent content in general?
| Photo (detail): © Adobe
From Online Safety to Total Erasure
There are numerous other examples: The erasure of sexual expression under the guise of “online safety” or the classification of mis- and disinformation are both largely conducted by AI, trained on datasets that are also based on inherently political policies. While for all of these examples the policies themselves are known, the rate of error in most cases is not. In other words, while we can dissect the policies and advocate for their change, it is impossible to see and therefore difficult to comprehend just how much legitimate expression (that is expression that falls outside the restrictions) is additionally captured and removed by AI with minimal if any oversight.
What, then, beyond understanding the political nature of and striving for transparency in the use of AI, are we to do? Are we to simply accept this as our new reality, or are there other interventions we can engage in to change the course of “progress”?
As I argue in my recent book, Silicon Values: The Future of Free Speech Under Surveillance Capitalism, the future remains ours to write. We must not simply accept this new zeitgeist as a given, and instead insist that, “decisions about what we are allowed to express should be given more human attention and more care, not handed to the whims of unaccountable actors and algorithms.”
This means, ultimately, that we must not merely seek to mitigate harms created by, but to reshape, rescale, and perhaps even dismantle these technological systems.